13. December 2023
Nvidia RTX 4090 vs. Apple M1 Pro with MLX: A Comparative Analysis
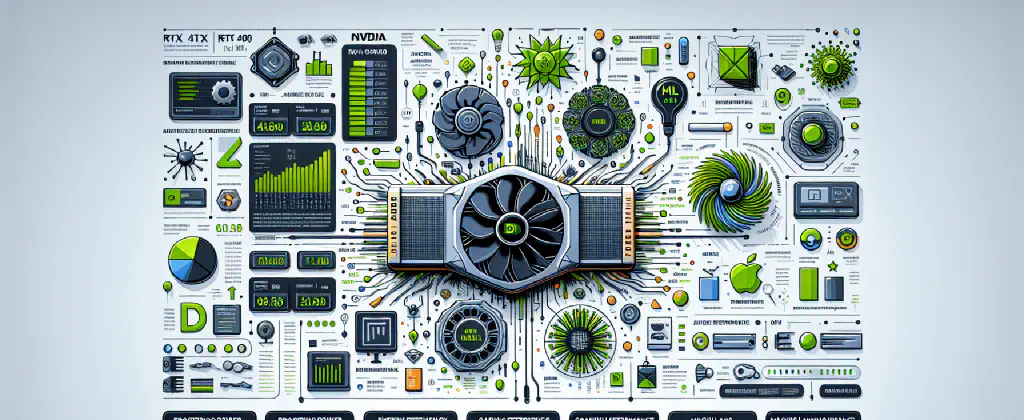
The battle between Nvidia’s RTX 4090 and Apple’s M1 Pro with MLX has sparked significant interest among machine learning enthusiasts. Both GPUs offer powerful capabilities, but it is important to carefully analyze and understand their performance in specific contexts. In this article, we will delve into key insights from user comments to provide an in-depth comparison between the Nvidia RTX 4090 and Apple M1 Pro with MLX.
The Challenge of Optimization
One user raised concerns about the optimization of software implementations, emphasizing the need to consider how well the software is optimized for a particular hardware platform. The inference difference between Nvidia GPUs and Apple M1 Max with respect to Llama and Stable Diffusion models was mentioned, highlighting the variation in performance across different model architectures.
The Whisper and SDXL Architecture
Comparisons between different models and architectures were discussed by users. It was noted that Whisper, an encoder-decoder transformer model, should not be directly compared to latent diffusion models like SDXL, as they have distinct architectures and purposes. Additionally, there was speculation about the optimizations specific to Apple Silicon on Whisper implementations.
Nvidia’s Optimization Advantage
A user pointed out that Nvidia has a history of highly optimized implementations, thanks to their dominant market position and extensive resources. Efforts to optimize implementations for other platforms, such as AMD, Intel, and Apple, are still in early stages compared to Nvidia’s long-standing optimization efforts. However, Apple’s unique approach of hand-writing their own Metal implementation for Stable Diffusion is worth mentioning.
The Role of MLX in Performance
MLX, Apple’s optimized machine learning framework, was brought into the discussion. Users questioned whether MLX was utilized in the previous tests conducted with Llama and Stable Diffusion models. MLX optimizations were considered to be a significant factor in the performance of Apple M1 Pro with MLX.
Memory Efficiency and Model Size
Another aspect that affects performance is memory efficiency and model size. While Nvidia GPUs excel in handling smaller models, the Nvidia RTX 4090 was praised for its memory efficiency, particularly with models up to 70B in size. However, it was noted that when comparing performance, the specific model size and quantization must be taken into account.
Apples and Oranges: The Challenge of Comparisons
One user highlighted the importance of considering the limitations and differences between the Nvidia RTX 4090 and Apple M1 Pro with MLX. While the M1 Max has a high memory bandwidth of 400GB/s and 32 GPU cores, the RTX 4090 boasts a memory bandwidth of 1TB/s and a staggering 16,000 cores. It is crucial to avoid direct comparisons between these GPUs due to the fundamental architectural and performance differences.
The Optimized Power of Nvidia and Apple’s MLX
The ongoing optimization efforts for Nvidia’s GPUs and Apple’s MLX were mentioned. Several projects are focused on bringing MLX-enabled backends to popular machine learning workloads. While inference workloads have seen notable acceleration, convolutional operations, commonly used in GANs and stable diffusion models, have not seen significant performance uplifts on MLX. This could be due to hardware limitations or unoptimized MLX libraries.
In conclusion, the performance comparison between the Nvidia RTX 4090 and Apple M1 Pro with MLX is a complex topic that requires careful consideration of various factors, including software optimization, model architectures, memory efficiency, and specific use cases. Both Nvidia and Apple continue to invest in optimizing their hardware and software for machine learning workloads. It is critical to understand the specific context and limitations of each GPU before making any definitive conclusions.